Georgia Tech Part of NASA Safe Aviation Autonomy Initiative
“Flying robots.”
Those two words encapsulate Panagiotis (Panos) Tsiotras’ work at Georgia Tech: enabling future electronic autonomous aircraft to fly and land safely.
A faculty member in the Daniel Guggenheim School of Aerospace Engineering, Tsiotras is part of a multi-institution team selected for a NASA University Leadership Initiative (ULI) focused on Safe Aviation Autonomy. The team is three years into a four-year project to build safe, efficient autonomous aviation systems. Georgia Tech joins researchers from both academia and industry, among them, Stanford, UC Berkeley, MIT, University of New Mexico, Raytheon, Hampton University, and MIT Lincoln Laboratory.
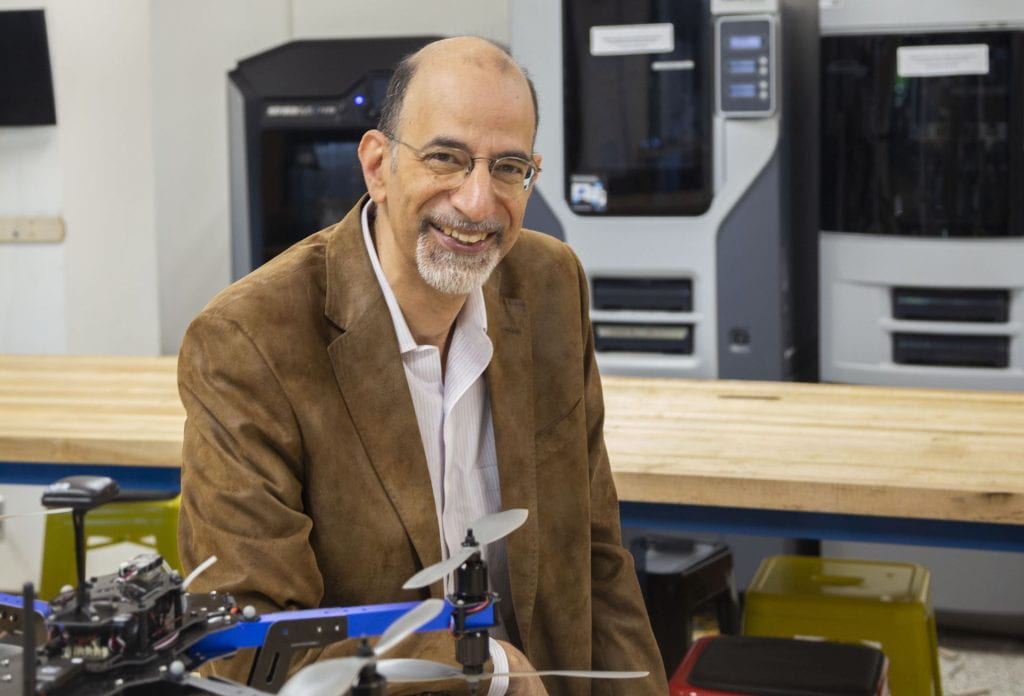
The flight mechanics & control expert hopes to make these future autonomously operated Urban Air Mobility (UAM) vehicles as “smart” as possible. His lab is focused on two areas of study: one, using Artificial Intelligence (AI) techniques to improve the aircraft’s ability to land reliably and safely even when faced with uncertainties; and two, improving the air traffic management system overseeing these autonomous vehicles, so the vehicles can maneuver without colliding into one another. It’s research that has huge stakes, compared to other autonomy advances in the consumer world, where brands like Amazon and Netflix use customer viewing and search data to power their recommendation engines.
“It’s one thing if the system makes a mistake and recommends the wrong film to you. That’s no big deal — the worst-case scenario is you spend a couple of hours watching something you don’t like. If something like this happens in an aircraft, people will die. So, it’s very important that these AI systems are robust, reliable and dependable,” Tsiotras says.
Take-offs and landings are the most critical, and riskiest maneuvers from a safety standpoint regardless of the aircraft. According to Tsiotras, those phases of flight present unique problems for low-flying Electric Vertical Take-Off and Landing vehicles (eVTOLs), which must launch from the top of a heliport on a skyscraper, avoiding other buildings or vehicles.
“The aircraft wanting to land may face constraints in its field of view. You must hit the landing zone accurately regardless of any environmental disturbances such as air gusts or unexpected [events] like sensor failures,” he says, adding that the system should be able to recognize an issue, compensate for it without interrupting the flight.
Autonomous drones are already flying today, delivering packages for Amazon and other retailers. The automotive industry is moving aggressively toward self-driving cars, sometimes with fatal consequences. But, putting these capabilities on aviation vehicles with passengers will take much longer to meet the aviation industry’s stringent safety requirements, the Georgia Tech researcher emphasizes.
Nevertheless, “there’s been tremendous increases in terms of these autonomous systems capabilities, primarily with AI decision making,” he says, citing Airbus demonstrating vision-only landing of a commercial aircraft a couple of years ago, and other major aviation manufacturers looking at these systems.
Autonomous systems in the near future will first serve as a backup system like an advanced level of autopilot, he predicts. Once pilots get used to the systems, they will gradually become more involved in on-board decision making.
“Today, the pilot gives the high-level commands such as cruising altitude and the autopilot will follow these commands. Now we’re discussing one level higher, where the commands themselves are taken by an autonomous system,” he says.
Tsiotras says his work shows that autonomous systems could perform better than humans in a future air traffic management system that manages flight paths and prevents collisions for thousands of eVTOLs.
“Machines excel at making decisions very fast if they have the right information, but are less capable when the situation is not as clear,” he says. “This is one of the bottlenecks.”
Specifically, machines are more susceptible to errors when they encounter a messy situation where it is not clear what is going on. In contrast, humans can often quickly understand the context of a situation, or environments that are structurally adaptable, but they can make mistakes out of fatigue, distraction or fear, which machines don’t experience.
“What is the right context and how you can teach the machine judgment — something that people gain by experience or common sense?” he questions.
In his research with NASA, Tsiotras is challenging these machines to make decisions even in very unstructured, very dynamic-involving environments. He won’t speculate on when autonomously operated urban air taxis will take over urban skylines — just that it can only happen once public confidence in the vehicles’ safety and reliability is assured.
“It’s not easy. Regardless of what you read in the news about autonomous vehicles around the corner, it’s going to take time, but we’re making progress slowly,” he concludes.